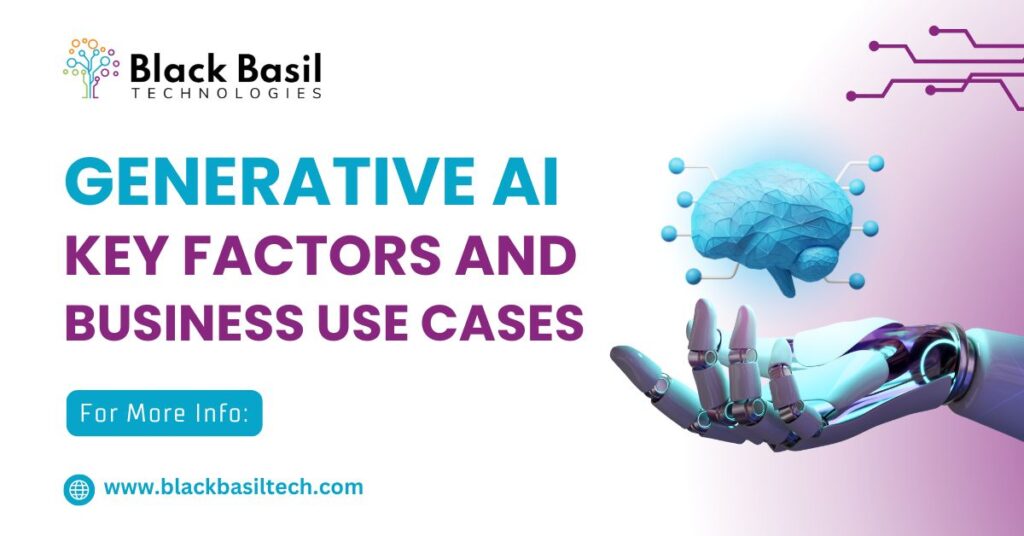
Generative AI Product Development: Key Factors and Business Use Cases
Generative AI has become a transformative force in various industries, offering innovative solutions that enable businesses to enhance efficiency, drive creativity, and achieve better outcomes. Developing generative AI products involves complex decision-making processes influenced by multiple factors that can impact their implementation in business use cases. This article provides a comprehensive analysis of the key considerations for generative AI product development, the tradeoffs involved, and the challenges faced in this field.
Understanding Generative AI
Generative AI refers to algorithms, primarily based on machine learning techniques, that can generate new data or content similar to the input data it has been trained on. These systems can create text, images, music, code, and even entire virtual environments. Techniques such as Generative Adversarial Networks (GANs), Variational Autoencoders (VAEs), and Transformer models (like GPT-3) are commonly used to develop generative AI products.
Key Factors in Generative AI Product Development
When building generative AI products, businesses need to consider several factors that can significantly influence the product’s development and its ultimate success in the market. Here are the most critical factors:
Data Quality and Quantity
The quality and quantity of data are fundamental to the performance of any generative AI model. These models rely on vast amounts of data to learn and generate new content that closely resembles the input data. High-quality, well-structured data enables the AI to create more accurate and meaningful outputs.
Tradeoff: While more data can lead to better model performance, it also increases computational costs and training times. Striking the right balance between data volume and data quality is essential to ensure that the AI model is efficient without being overly resource-intensive.
Tradeoff: While more data can lead to better model performance, it also increases computational costs and training times. Striking the right balance between data volume and data quality is essential to ensure that the AI model is efficient without being overly resource-intensive.
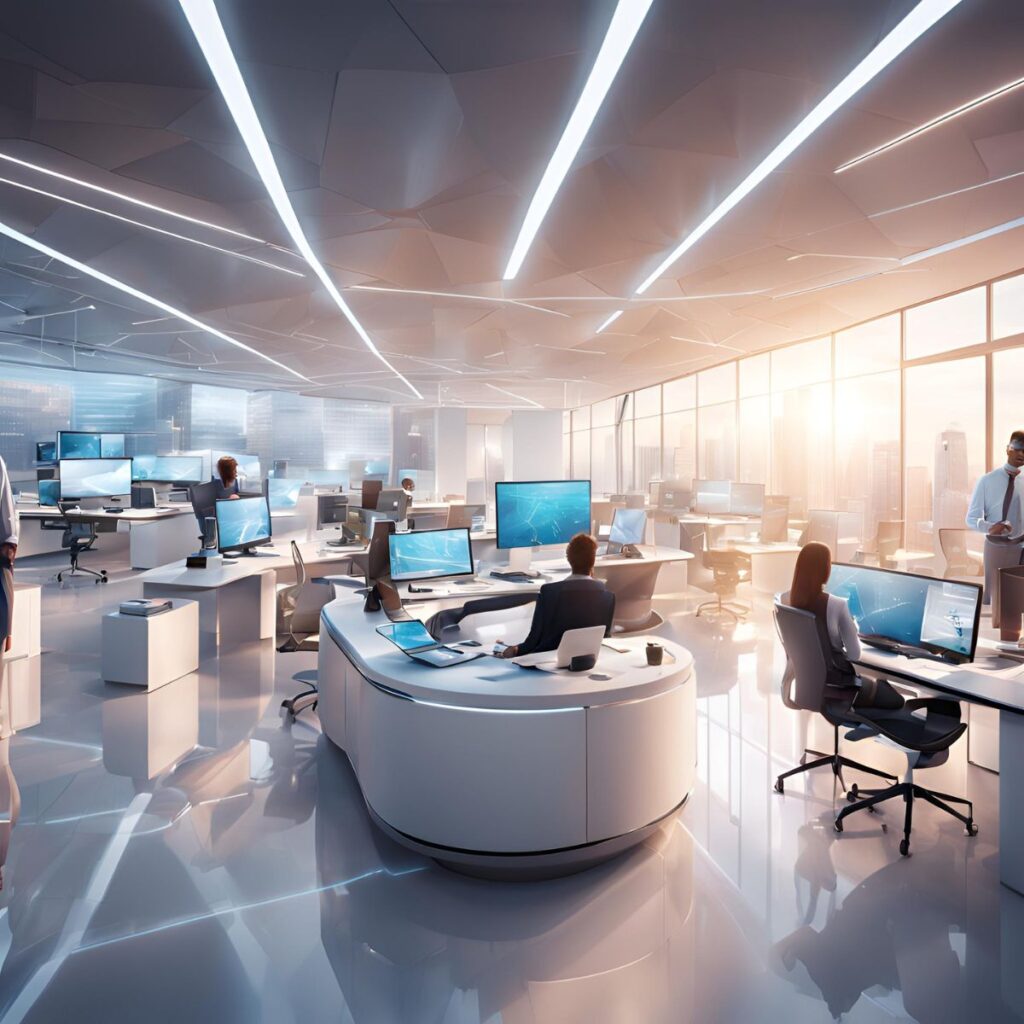
Algorithm Selection
Choosing the right algorithm is crucial in generative AI product development. Different algorithms have unique strengths and limitations depending on the desired output. For instance, GANs are particularly suited for generating realistic images, while transformer models are excellent at producing human-like text.
Tradeoff: The challenge lies in balancing the algorithm’s complexity with the processing power required. More advanced algorithms can produce better results but may also demand higher computational resources, which can be costly and time-consuming.
Tradeoff: The challenge lies in balancing the algorithm’s complexity with the processing power required. More advanced algorithms can produce better results but may also demand higher computational resources, which can be costly and time-consuming.
Computational Power and Infrastructure
Generative AI requires significant computational power to train and run models effectively. High-performance hardware, such as GPUs and TPUs, and robust cloud infrastructure are often necessary to handle the computational demands.
Tradeoff: Investing in high-end infrastructure can improve AI model performance but may lead to increased operational costs. Businesses need to weigh the benefits of faster, more accurate outputs against the expenses of maintaining powerful hardware and cloud solutions.
Tradeoff: Investing in high-end infrastructure can improve AI model performance but may lead to increased operational costs. Businesses need to weigh the benefits of faster, more accurate outputs against the expenses of maintaining powerful hardware and cloud solutions.
Ethical Considerations and Bias
AI-generated content can sometimes reflect biases present in the training data, leading to potentially unfair or unethical outcomes. It is crucial to design generative AI systems that are transparent, unbiased, and aligned with ethical standards.
Tradeoff: Balancing accuracy with fairness is a significant challenge in generative AI. While removing bias might affect the precision of the AI’s outputs, it is essential to prioritize ethical considerations to avoid unintended consequences.
Tradeoff: Balancing accuracy with fairness is a significant challenge in generative AI. While removing bias might affect the precision of the AI’s outputs, it is essential to prioritize ethical considerations to avoid unintended consequences.
User Experience and Human-AI Interaction
The effectiveness of generative AI products also depends on how users interact with the AI system. Designing user-friendly interfaces and intuitive workflows is critical to ensure that the AI product adds value to the user experience.
Tradeoff: Enhancing user experience may require simplifying the AI’s functionality, which could limit its capabilities. The key is to find a balance between ease of use and the complexity of features offered by the AI.
Tradeoff: Enhancing user experience may require simplifying the AI’s functionality, which could limit its capabilities. The key is to find a balance between ease of use and the complexity of features offered by the AI.
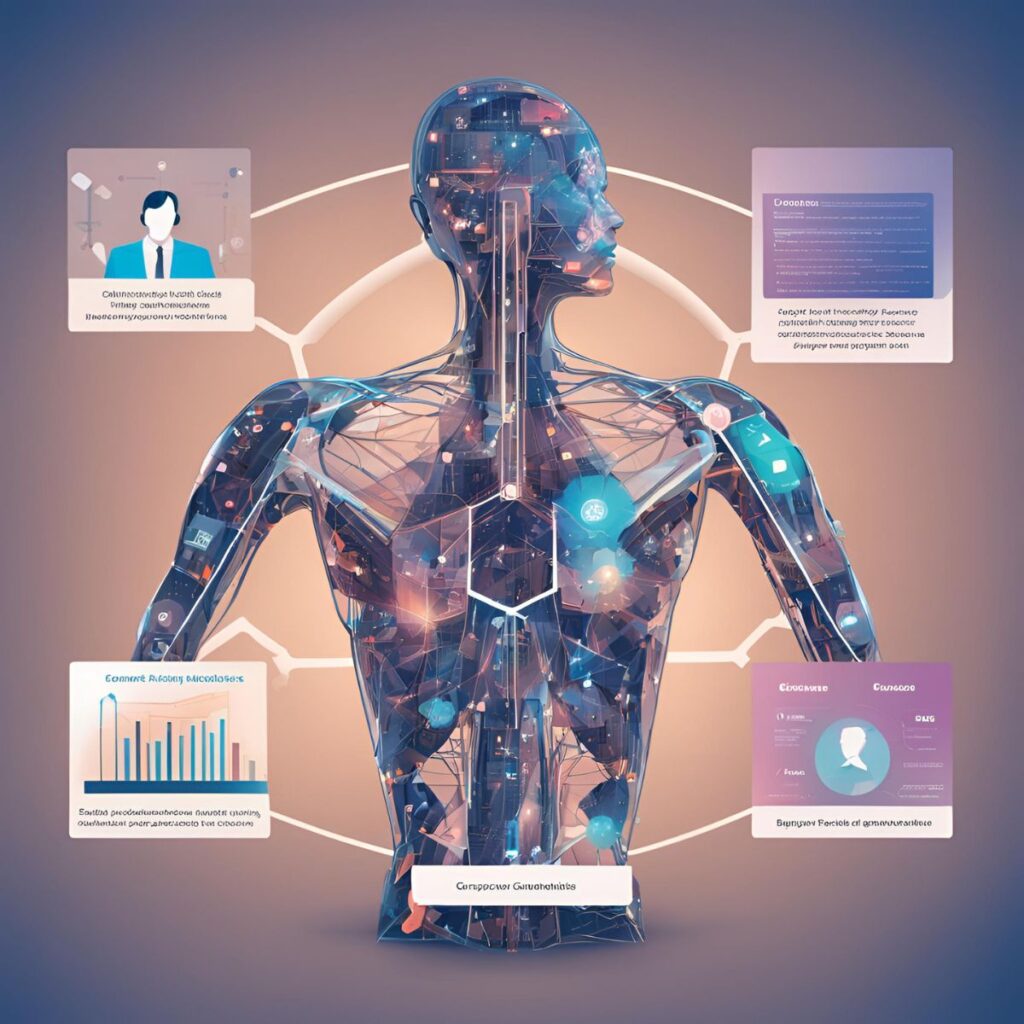
User Experience and Human-AI Interaction
Generative AI has a wide range of applications in business. Here are some of the most prominent use cases:
Content Creation and Marketing
Generative AI can automate content creation for blogs, social media, email campaigns, and advertisements. It can help marketers generate personalized content at scale, saving time and resources.
Product Design and Prototyping
In industries like fashion, automotive, and architecture, generative AI is used to create new designs and prototypes. AI-driven models can generate multiple variations of a design, accelerating the product development cycle.
Healthcare and Drug Discovery
Generative AI is revolutionizing healthcare by identifying new drug compounds and predicting molecular structures. It speeds up the drug discovery process, helping pharmaceutical companies bring innovative medicines to market faster.
Customer Service and Chatbots
AI-powered chatbots and virtual assistants can engage with customers in a more personalized manner. Generative AI enables these systems to provide human-like responses, enhancing customer support and satisfaction.
Gaming and Entertainment
Generative AI is widely used in creating realistic game environments, characters, and storyline developments. It allows game developers to create immersive experiences with minimal manual intervention.
Challenges in Generative AI Product Development
Despite its potential, generative AI product development is not without challenges. Some of the primary obstacles include:
1. Data Privacy and Security: Handling sensitive data is a major concern, especially in industries like healthcare and finance. Ensuring data privacy and security while training AI models is critical to avoid potential breaches and compliance issues.
2. Scalability Issues: Scaling generative AI models to handle large datasets and extensive user interactions can be complex and costly. Businesses often struggle with maintaining model efficiency when dealing with increased workloads.
3. Model Interpretability Understanding :how generative AI models make decisions can be challenging, particularly with complex architectures like deep learning models. Lack of interpretability can hinder the trust and adoption of AI-driven solutions.
4. Regulatory Compliance: Navigating the regulatory landscape for AI technologies can be difficult, as laws and guidelines are still evolving. Companies must ensure that their generative AI products comply with industry standards and data protection regulations.
1. Data Privacy and Security: Handling sensitive data is a major concern, especially in industries like healthcare and finance. Ensuring data privacy and security while training AI models is critical to avoid potential breaches and compliance issues.
2. Scalability Issues: Scaling generative AI models to handle large datasets and extensive user interactions can be complex and costly. Businesses often struggle with maintaining model efficiency when dealing with increased workloads.
3. Model Interpretability Understanding :how generative AI models make decisions can be challenging, particularly with complex architectures like deep learning models. Lack of interpretability can hinder the trust and adoption of AI-driven solutions.
4. Regulatory Compliance: Navigating the regulatory landscape for AI technologies can be difficult, as laws and guidelines are still evolving. Companies must ensure that their generative AI products comply with industry standards and data protection regulations.
Importance of Considering the Impact
In generative AI product development, it is crucial to consider the broader impact of these technologies. Businesses need to evaluate how their AI solutions will affect end-users, the environment, and society at large. Responsible AI practices, including ethical design, transparency, and fairness, should be integrated into the development process to create AI products that not only meet business objectives but also benefit society.
Final Thoughts
Generative AI product development is a complex process that requires careful consideration of multiple factors, including data quality, algorithm selection, computational power, and ethical implications. By understanding these key elements and addressing the tradeoffs and challenges involved, businesses can unlock the full potential of generative AI to create innovative solutions for various use cases.
The success of generative AI in business lies in finding the right balance between technological advancements, ethical considerations, and practical implementations. As AI technologies continue to evolve, companies must remain adaptable and prioritize responsible AI practices to stay ahead in the competitive landscape.
The success of generative AI in business lies in finding the right balance between technological advancements, ethical considerations, and practical implementations. As AI technologies continue to evolve, companies must remain adaptable and prioritize responsible AI practices to stay ahead in the competitive landscape.
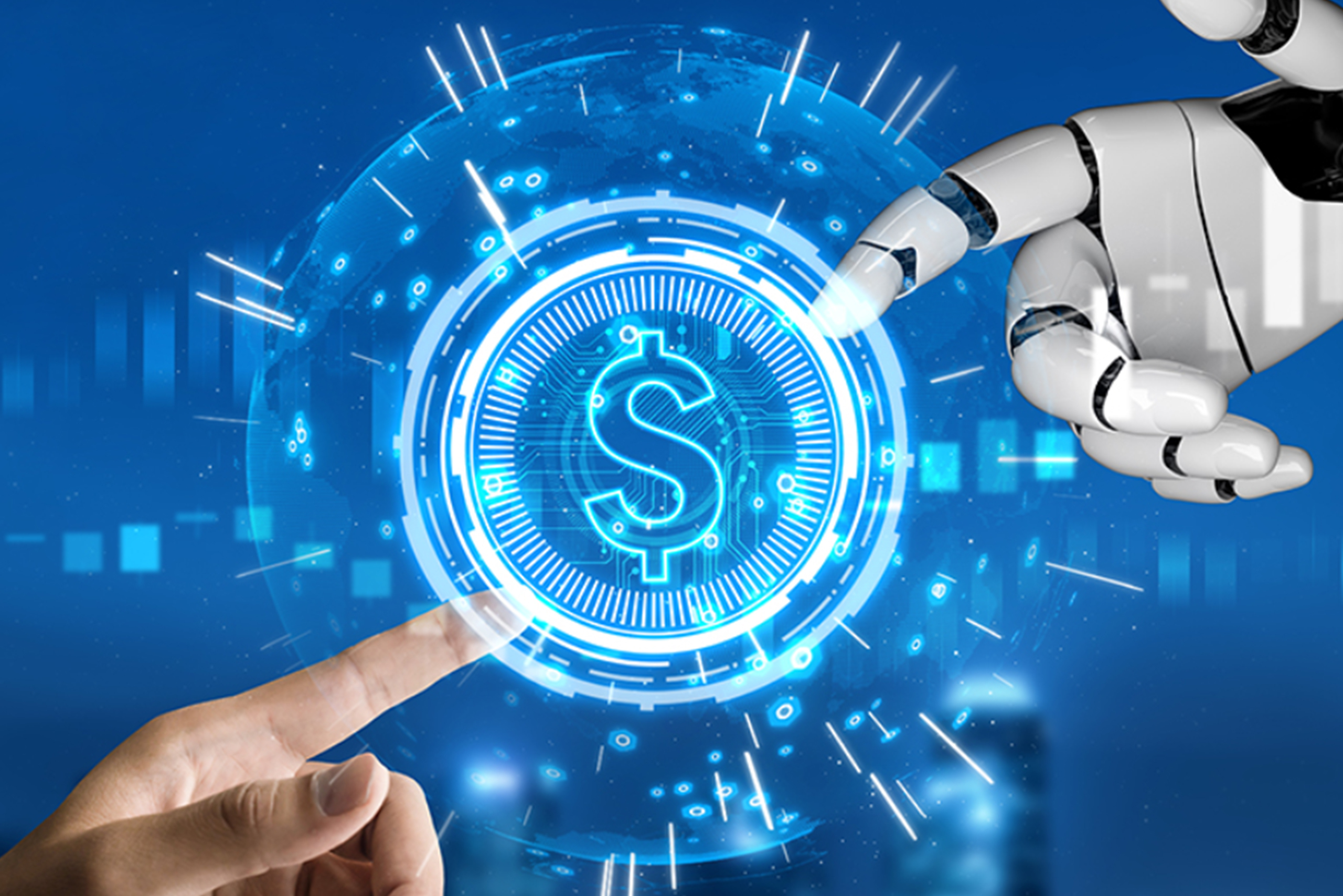
Hello